Pattern Generation#
Description: Pattern generation example with amplpy
Tags: amplpy, example
Notebook author: Filipe Brandão <fdabrandao@gmail.com>
Model author: N/A
References: N/A
# Install dependencies
%pip install -q amplpy matplotlib numpy
# Google Colab & Kaggle integration
from amplpy import AMPL, ampl_notebook
ampl = ampl_notebook(
modules=["gurobi"], # modules to install
license_uuid="default", # license to use
) # instantiate AMPL object and register magics
Basic pattern-cutting model#
%%ampl_eval
param nPatterns integer > 0;
set PATTERNS = 1..nPatterns; # patterns
set WIDTHS; # finished widths
param order {WIDTHS} >= 0; # rolls of width j ordered
param overrun; # permitted overrun on any width
param rolls {WIDTHS,PATTERNS} >= 0 default 0; # rolls of width i in pattern j
var Cut {PATTERNS} integer >= 0; # raw rolls to cut in each pattern
minimize TotalRawRolls: sum {p in PATTERNS} Cut[p];
subject to FinishedRollLimits {w in WIDTHS}:
order[w] <= sum {p in PATTERNS} rolls[w,p] * Cut[p] <= order[w] + overrun;
Plotting routine#
def cuttingPlot(roll_width, widths, solution):
import numpy as np
import matplotlib.pyplot as plt
ind = np.arange(len(solution))
acc = [0] * len(solution)
for p, (patt, rep) in enumerate(solution):
for i in range(len(widths)):
for j in range(patt[i]):
vec = [0] * len(solution)
vec[p] = widths[i]
plt.bar(ind, vec, width=0.35, bottom=acc)
acc[p] += widths[i]
plt.title("Solution")
plt.xticks(ind, tuple("x {:}".format(rep) for patt, rep in solution))
plt.yticks(np.arange(0, roll_width, 10))
plt.show()
Set data#
roll_width = 64.5
overrun = 6
orders = {6.77: 10, 7.56: 40, 17.46: 33, 18.76: 10}
widths = list(sorted(orders.keys(), reverse=True))
Send data to AMPL (Java/C++ style)#
from math import floor
# Send scalar values
ampl.get_parameter("overrun").set(overrun)
ampl.get_parameter("nPatterns").set(len(widths))
# Send order vector
ampl.get_set("WIDTHS").set_values(widths)
ampl.get_parameter("order").set_values(orders)
# Generate and send initial pattern matrix
ampl.get_parameter("rolls").set_values(
{(widths[i], 1 + i): int(floor(roll_width / widths[i])) for i in range(len(widths))}
)
Send data to AMPL (alternative style)#
# Send scalar values
ampl.param["overrun"] = overrun
ampl.param["nPatterns"] = len(widths)
# Send order vector
ampl.set["WIDTHS"] = widths
ampl.param["order"] = orders
# Generate and send initial pattern matrix
ampl.param["rolls"] = {
(widths[i], 1 + i): int(floor(roll_width / widths[i])) for i in range(len(widths))
}
Set up for generation loop#
# Set solve options
ampl.option["relax_integrality"] = 1
# Create a param for sending AMPL new patterns
ampl.eval("param newpat {WIDTHS} integer >= 0;")
new_pattern = ampl.param["newpat"]
Define the knapsack subproblem#
# Define the knapsack subproblem
subprob = AMPL()
subprob.eval(
"""
set WIDTHS;
param W >= 0;
param v{WIDTHS} >= 0;
var x{WIDTHS} integer >= 0;
maximize profit: sum {w in WIDTHS} v[w]*x[w];
subject to capacity: sum {w in WIDTHS} w*x[w] <= W;
"""
)
subprob.set["WIDTHS"] = widths
subprob.param["W"] = roll_width
values = subprob.param["v"]
kpsolution = subprob.var["x"]
profit = subprob.obj["profit"]
Loop#
limits = ampl.get_constraint("FinishedRollLimits")
while True:
print("Master problem:")
ampl.solve(solver="gurobi")
assert ampl.solve_result == "solved", ampl.solve_result
# Retrieve duals & look for new pattern
# Solve knapsack problem for potential new pattern
values.set_values(limits.get_values())
print("\nSubproblem:")
subprob.solve(solver="gurobi")
assert subprob.solve_result == "solved", subprob.solve_result
print()
if profit.value() <= 1.000001:
break
# Send new pattern to AMPL
new_pattern.set_values(kpsolution.get_values())
ampl.eval("let nPatterns := nPatterns + 1;")
ampl.eval("let {w in WIDTHS} rolls[w, nPatterns] := newpat[w];")
Master problem:
Gurobi 9.5.0: optimal solution; objective 20.44444444
Subproblem:
Gurobi 9.5.0: optimal solution; objective 1.152777778
1 simplex iterations
1 branch-and-cut nodes
Master problem:
Gurobi 9.5.0: optimal solution; objective 18.77777778
1 simplex iterations
Subproblem:
Gurobi 9.5.0: optimal solution; objective 1.111111111
Master problem:
Gurobi 9.5.0: optimal solution; objective 18.375
3 simplex iterations
Subproblem:
Gurobi 9.5.0: optimal solution; objective 1.125
1 simplex iterations
1 branch-and-cut nodes
Master problem:
Gurobi 9.5.0: optimal solution; objective 17.95833333
5 simplex iterations
Subproblem:
Gurobi 9.5.0: optimal solution; objective 1.041666667
2 simplex iterations
1 branch-and-cut nodes
Master problem:
Gurobi 9.5.0: optimal solution; objective 17.94117647
5 simplex iterations
Subproblem:
Gurobi 9.5.0: optimal solution; objective 1
1 simplex iterations
1 branch-and-cut nodes
Compute and display integer solution#
# Compute and display integer solution
ampl.option["relax_integrality"] = 0
ampl.solve(solver="gurobi")
assert ampl.solve_result == "solved", ampl.solve_result
# Retrieve solution
cutvec = ampl.var["Cut"].to_list(skip_index=True)
# Display solution
rolls = ampl.param["rolls"]
npatterns = int(ampl.param["nPatterns"].value())
solution = [
([int(rolls[widths[i], p]) for i in range(len(widths))], int(cutvec[p]))
for p in range(npatterns)
if cutvec[p] > 0
]
cuttingPlot(roll_width, widths, solution)
Gurobi 9.5.0: optimal solution; objective 19
5 simplex iterations
1 branch-and-cut nodes
[0.0, 0.0, 0.0, 0.0, 10.0, 4.0, 4.0, 1.0]
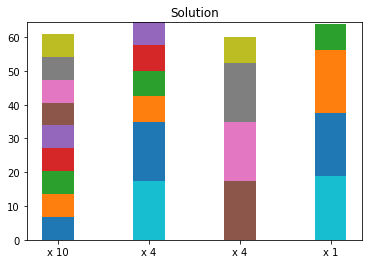